This image was created by and is owned by the contributors, Pinscreen and University of Southern California Institute for Creative Technologies.
During the SIGGRAPH 2020 Real-Time Live! showcase, many demonstrations stood out to the live, virtual audience, but “Volumetric Human Teleportation” lay above the rest and ultimately tied for the coveted, jury-selected Best in Show award, sharing the honor with “Interactive Style Transfer to Live Video Streams”.
For the demo, the team behind “Teleportation” introduced the first system that can capture a completely clothed human body (including the back) using a single RGB webcam … in real time. The deep-learning-based approach enables new possibilities for low-cost and consumer-accessible immersive teleportation, and hails from University of Southern California Institute for Creative Technologies (USC ICT) and Pinscreen team members Zeng Huang, Hao Li, Ruilong Li, Kyle Olszewski, Shunsuke Saito, and Yuliang Xiu. Catch the full demo starting at 53:45 on the ACM SIGGRAPH YouTube channel. We caught up with the Hao Li and Ruilong Li to learn more about the technology and its future applications.
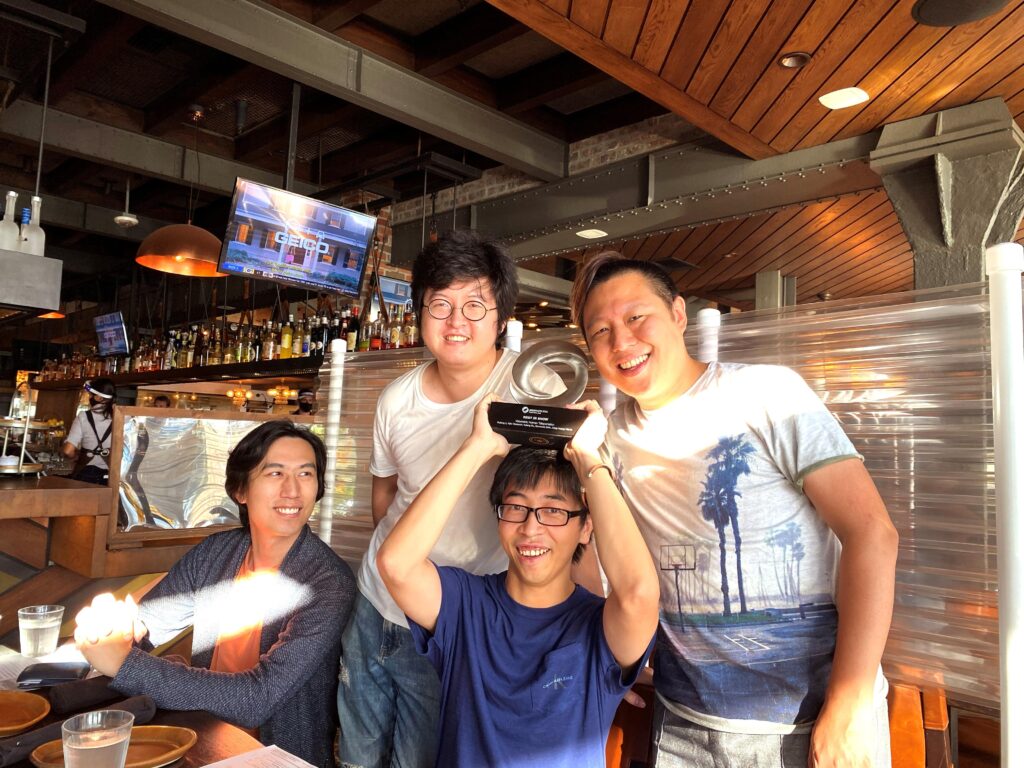
SIGGRAPH: “Volumetric Human Teleportation” was a partnership between USC and Pinscreen. Share a bit about the background of this project. What inspired the concept?
Hao and Ruilong (H&R): From a research standpoint, we have always been focusing on advancing new technological capabilities around digital humans and their applications for AR- and/or VR-based telepresence, immersive content creation, and entertainment. While digital characters are everywhere in visual effects and video games, the ability for anyone to create their personal [characters] without the need of a production studio has always been our goal.
We are exploring two ways of digitizing personalized virtual avatars. One way is to build photoreal and customizable parametric models of humans from images or videos, which is what we productize at Pinscreen. The other approach is to capture a person in a scene directly using volumetric capture. For telepresence applications (examples include Microsoft’s holoportation system), existing systems always need a capture environment with multiple cameras and/or 3D sensors that are placed around a person, and this exactly is the barrier to entry for consumer accessibility. It is unlikely that people will have a multi-view capture system at home, so we thought, “Why not use 3D deep learning to predict a full 3D model of a person with only a single view as input?”
Two years ago, we started working on a several methods for inferring complete, 3D-clothed humans from a single photo, and we came up with an approach called PIFu, which stands for Pixel-aligned Implicit Functions. The method could generate pretty decent textured 3D models from a single image, but the computation still took roughly one minute per frame. For telepresence applications, the next challenge was to come up with a technique that can achieve real-time performance, which led to this research project of “Volumetric Human Teleportation”.
SIGGRAPH: When executing the idea, talk about the challenges faced and overcome. What was most difficult to produce? Research? How did the teams work together?
H&R: This project was the result of multiple years of research, which included early findings on how to generate high-fidelity textured 3D models of a complete person, including its clothing, from a single-input image. The foundations were developed through active and intensive collaborations between USC ICT, Pinscreen, Waseda University, and UC Berkeley, which has led to our seminal work called PIFu.
For the Real-Time Live! demonstration, the most challenging aspect was to achieve real-time capabilities for a computation that takes roughly one minute per frame. This meant we needed to speed up the processing by three orders of magnitude, without sacrificing the quality. Even when using the fastest GPUs, we wouldn’t be able to achieve this. Consequently, we split our problem into two parts, one that focused on developing a new algorithm based on a new octree-based representation to accelerate the surface sampling and rendering process, and one that focuses on engineering a novel, deep learning-based inference framework that can schedule tasks efficiently on multiple GPUs. With the help of two Nvidia GV100 GPUs we were able to achieve real-time performance for digitizing and rendering a complete model of a clothed subject including its texture.
SIGGRAPH: How were you able to go from multiple calibrated cameras to just one web-based camera for the real-time functionality of “Volumetric Human Teleportation”?
H&R: Existing volumetric capture systems use calibrated sensors and methods such as multi-view stereo, depth sensors, or visual hull to obtain the full 3D model. Our volumetric human teleportation is based on PIFu, which is a 3D deep-learning method that we developed last year to generate a complete 3D-clothed human model from a single unconstrained photo.
Compared to our original method, our Real-Time Live! demo was done in real time through an accelerated algorithm and a highly optimized multi-GPU deep neural network inference framework. To obtain a complete 3D model from a single photo, one has to be able to predict what the shape and texture looks like for unseen views, which is whatever is occluded and not visible from the current view. Our 3D, deep-learning approach consists of predicting the spatial occupancy and color of the surface using a deep neural network. That network is trained with hundreds of photogrammetry scans of clothed people. When sufficient data is provided, the network not only predicts the depth of every pixel, but can also predict how the back of the person looks, including its texture. One key aspect is the introduction of our highly effective data representation based on pixel-aligned implicit functions (also PIFu), which allows us to produce high resolution textured meshes.
SIGGRAPH: Explain the ways deep learning technology was used on this project.
H&R: Conventional volumetric capture systems use multiple sensors around the subject to obtain the full 3D model and to cover as much surface as possible. If our goal is to predict an entire clothed human body, including its texture. We will need to predict and infer both the surface’s geometry and colors. We use 3D deep learning combined with a pixel-aligned implicit function representation to determine if at a certain point in space we are inside or outside the surface. Using our lossless octree-based data structure we can sample sufficient points in real-time so that we can extract an isosurface and predict the visible part from an arbitrary view together with the surface colors. In particular, we train our network with a large number of photogrammetry scans that are rendered from all possible angles.
SIGGRAPH: What’s next for “Teleportation”? Any additional developments or enhancements planned?
H&R: While our prototype demonstrates that it is possible to predict a 3D-clothed human body performance from an arbitrary viewpoint, the ability to handle multiple people and even accessories, such as bags and clothing parts, our current system only renders and streams the desired viewpoint. Even though we can show how the subject can be integrated inside a virtual environment, it is only suitable for free viewpoint applications based on a single view such as tablet AR applications.
Ultimately, we would like to use it to stream into virtual worlds that can be seen with binocular displays or even with multiple users. Hence, we would need to develop an approach that can extract the full 3D-textured model and stream this directly into a 3D virtual environment. In addition to this, we are also interested in developing a method that can generate relightable volumetric captures of people. One way would be to normalize its lighting condition even though it is captured in an unconstrained environment. Last but not least, we want to further increase the fidelity and texture quality of the captured subjects while still retaining real-time capabilities. A recent work called PIFuHD, by one of our co-authors, demonstrates that it is possible to generate higher fidelity geometries using a multi-resolution inference approach.
SIGGRAPH: Share your favorite SIGGRAPH memory.
H&R: All of us had attended SIGGRAPH before 2020, and many of us had presented at the conference already. While the Technical Papers sessions are important for us researchers, Real-Time Live! is certainly one of the most memorable events, as we can witness some of the most impressive live demos with a huge audience, and see what is cutting edge in both industry and academia. We certainly miss the physical SIGGRAPH conference, but the virtual conference this year did not disappoint. It has been a dream for us to continuously participate and contribute to technological breakthroughs at SIGGRAPH, not to mention to have received the “Best in Show” award.
SIGGRAPH: What advice do you have for someone who wants to submit to Real-Time Live! for a future SIGGRAPH conference?
H&R: Our teams at both Pinscreen and USC ICT had the chance to have been selected eight times for Real-Time Live! over the past few years. Each time, we have to think about a new idea combined with new technological advances, and, most importantly, a demo that can impress the audience. The most exciting part is to compete with big players in the industry as well as top research groups in academia.
The biggest difference between submitting a Real-Time Live! demo and, for example, a Technical Paper, is that, for Real-Time Live!, the 6-minute impression is your key to success. The demo must speak for itself, and it doesn’t necessarily have to be a scientific output. It can be an engineering masterpiece, a highly artistic demo, or a cutting-edge research demo. If it’s a fresh, new idea, a relevant problem, or just a jaw-dropping cool demo, as long as it is well polished, entertaining, and well executed, the submission will have a good chance. I would definitely look at past years’ Real-Time Live! demos and explore the breadth of types of submissions and the high-quality level of presentations. I would also encourage researchers who submit research papers (graphics, vision, or AI communities) with a real-time component to it and impressive graphics, to consider a submission to Real-Time Live!.
Over 250 hours of SIGGRAPH 2020 content is available on-demand through 27 October. Learn more.
Meet the Contributors
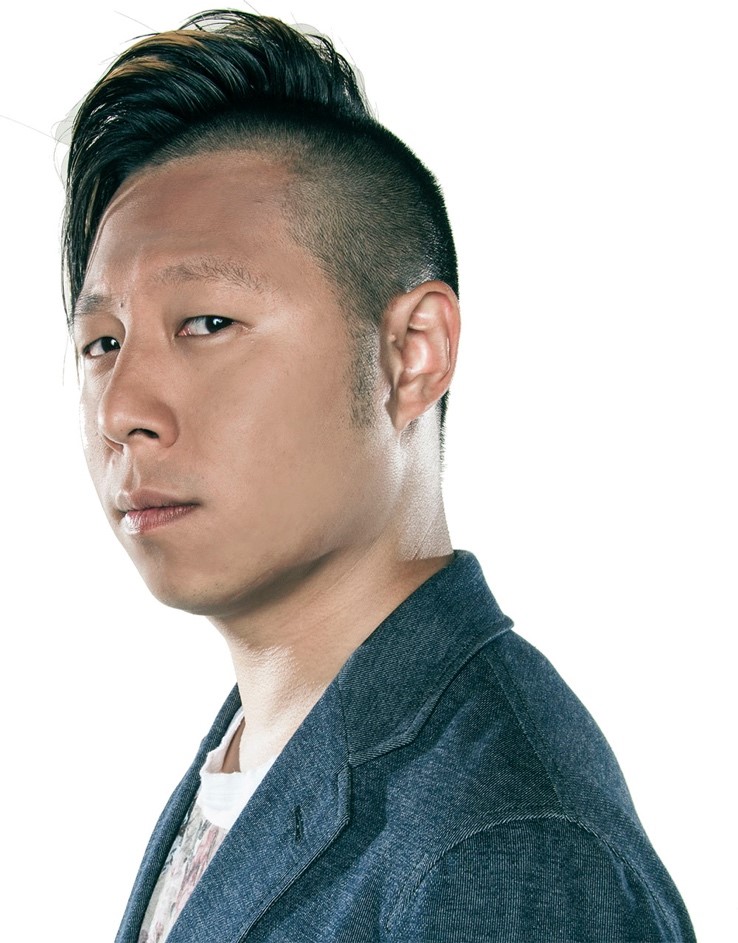
Hao Li is CEO and co-Founder of Pinscreen, a startup that builds cutting edge AI-driven virtual avatars. Before that, he was an Associate Professor of Computer Science at the University of Southern California, as well as the director of the Vision and Graphics Lab at the USC Institute for Creative Technologies. Hao’s work in Computer Graphics and Computer Vision focuses on human digitization and performance capture for immersive telepresence, virtual assistants, and entertainment. His research involves the development of noveldeep learning, data-driven, and geometry processing algorithms. He is known for his seminal work in avatar creation, facial animation, hair digitization, dynamic shape processing, as well as his recent efforts in preventing the spread of malicious deep fakes. He was previously a visiting professor at Weta Digital, a research lead at Industrial Light & Magic / Lucasfilm, and a postdoctoral fellow at Columbia and Princeton Universities. He was named top 35 innovator under 35 by MIT Technology Review in 2013 and was also awarded the Google Faculty Award, the Okawa Foundation Research Grant, as well as the Andrew and Erna Viterbi Early Career Chair. He won the Office of Naval Research (ONR) Young Investigator Award in 2018 and was named to the DARPA ISAT Study Group in 2019. Hao obtained his PhD at ETH Zurich and his MSc at the University of Karlsruhe (TH).
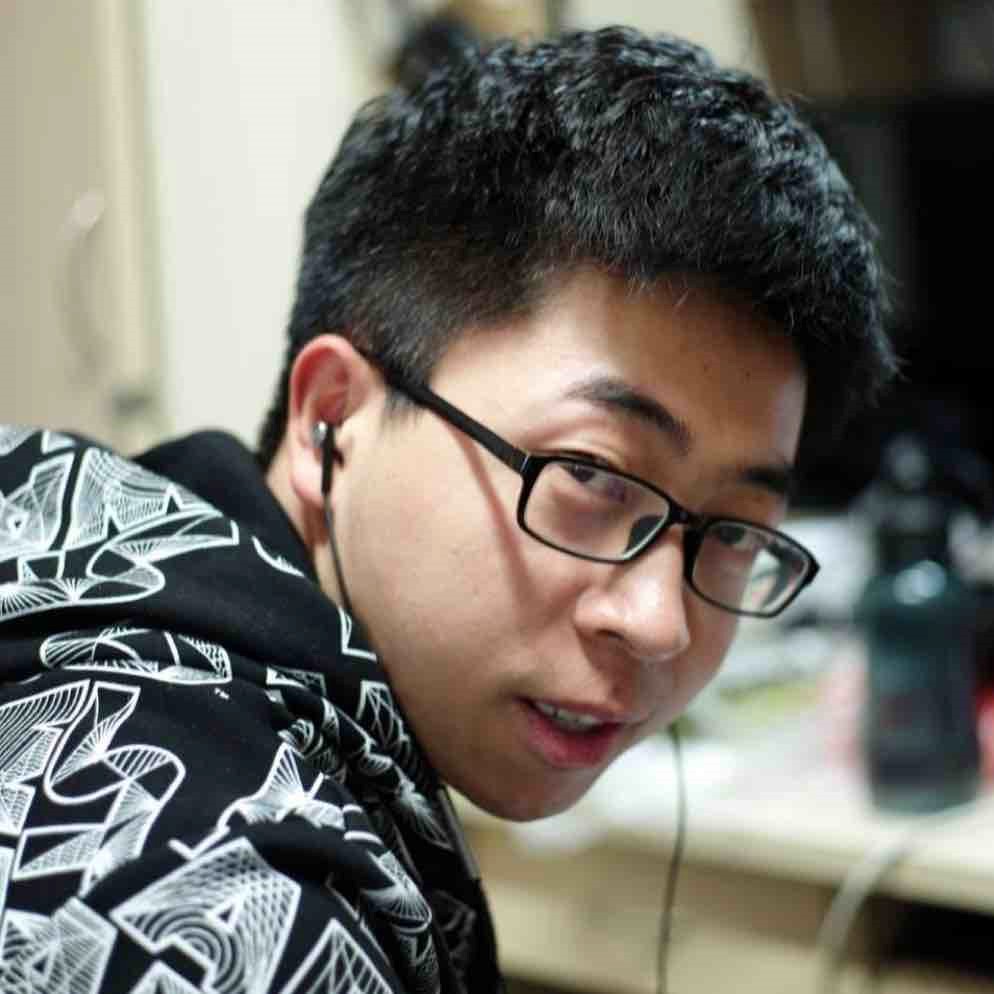
Ruilong Li is a second-year Ph.D. student at the University of Southern California, advised by Hao Li. His research lies at the intersection field of Computer Vision and Graphics, with focus on developing new synthesis and digitization methods from images. He has published extensively at top vision conferences including 3 CVPR and 1 ECCV papers, and interned at Google Research in 2020 working on autonomous motion synthesis with Shan Yang and Angjoo Kanazawa. Ruilong received his BSc degree in Physics and Mathematics, as well as his MSc degree in Computer Science, both from Tsinghua University.